|
ACCESS THE FULL ARTICLE
No SPIE Account? Create one
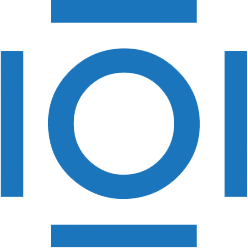
CITATIONS
Cited by 1 scholarly publication.
Fuzzy logic
Magnetic resonance imaging
Image segmentation
Data modeling
Neck
Machine learning
Nonuniformity corrections