|
ACCESS THE FULL ARTICLE
No SPIE Account? Create one
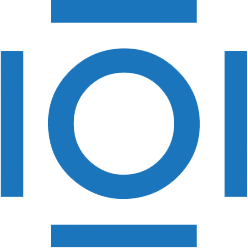
CITATIONS
Cited by 31 scholarly publications.
Machine learning
Acoustics
Ultrasonography
Signal detection
Wavefronts
Convolutional neural networks
Image resolution