|
ACCESS THE FULL ARTICLE
No SPIE Account? Create one
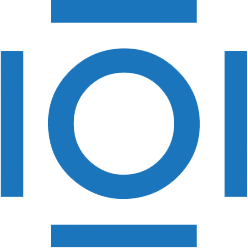
CITATIONS
Cited by 8 scholarly publications.
Computed tomography
Lung
Computer aided diagnosis and therapy
CAD systems
Databases
Data analysis
Artificial neural networks