|
ACCESS THE FULL ARTICLE
No SPIE Account? Create one
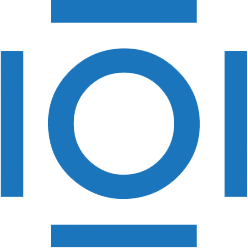
CITATIONS
Cited by 1 scholarly publication.
Remote sensing
Associative arrays
Data modeling
Statistical analysis
Environmental sensing
Image classification
Sensors