|
ACCESS THE FULL ARTICLE
No SPIE Account? Create one
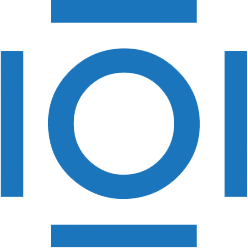
CITATIONS
Cited by 3 scholarly publications.
Matrices
Computer simulations
Error analysis
Data modeling
Image classification
Optical character recognition
Electronic imaging