|
ACCESS THE FULL ARTICLE
No SPIE Account? Create one
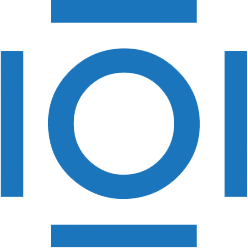
CITATIONS
Cited by 9 scholarly publications.
Fuzzy logic
Computing systems
Data modeling
Neural networks
Neurons
Algorithms
Artificial neural networks