|
ACCESS THE FULL ARTICLE
No SPIE Account? Create one
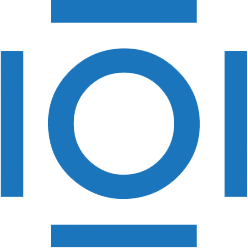
CITATIONS
Cited by 1 scholarly publication.
Raster graphics
Detection and tracking algorithms
Optical character recognition
Binary data
Image classification
Algorithm development
Antimony