|
ACCESS THE FULL ARTICLE
No SPIE Account? Create one
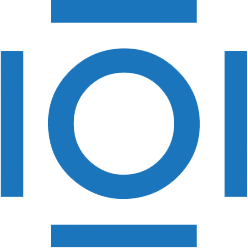
CITATIONS
Cited by 1 scholarly publication.
Image classification
Earth observing sensors
Landsat
Artificial neural networks
Error analysis
Vegetation
Data acquisition