|
ACCESS THE FULL ARTICLE
No SPIE Account? Create one
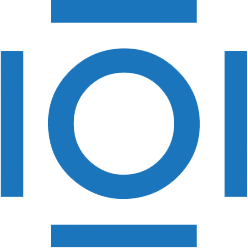
CITATIONS
Cited by 3 scholarly publications and 2 patents.
Cervical cancer
Data modeling
Image classification
Cancer
Classification systems
Tumor growth modeling
Neural networks