|
ACCESS THE FULL ARTICLE
No SPIE Account? Create one
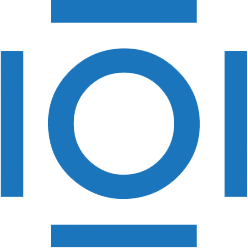
CITATIONS
Cited by 2 scholarly publications.
Feature extraction
Statistical analysis
Defect detection
Neural networks
Data modeling
Probability theory
Statistical modeling