|
ACCESS THE FULL ARTICLE
No SPIE Account? Create one
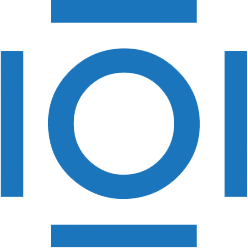
CITATIONS
Cited by 2 scholarly publications.
Neural networks
Quantization
Computer architecture
Convolution
Data storage
Image classification
Video surveillance