|
ACCESS THE FULL ARTICLE
No SPIE Account? Create one
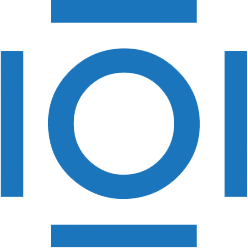
CITATIONS
Cited by 3 scholarly publications.
Fuzzy logic
Principal component analysis
Neural networks
Associative arrays
Satellites
Sensor fusion
Solids