|
ACCESS THE FULL ARTICLE
No SPIE Account? Create one
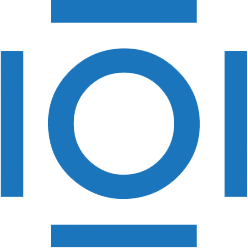
CITATIONS
Cited by 1 scholarly publication.
Control systems
Actuators
Expectation maximization algorithms
Neurons
Control systems design
Stochastic processes
Algorithm development