|
ACCESS THE FULL ARTICLE
No SPIE Account? Create one
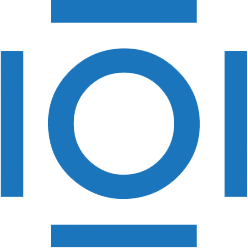
CITATIONS
Cited by 10 scholarly publications.
Data modeling
Tomography
Reconstruction algorithms
Statistical analysis
Monte Carlo methods
Medical imaging
Visual process modeling