|
ACCESS THE FULL ARTICLE
No SPIE Account? Create one
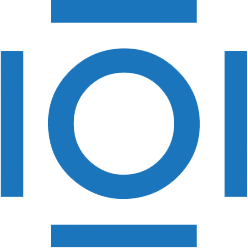
CITATIONS
Cited by 1 scholarly publication.
Breast cancer
Databases
Artificial neural networks
Mammography
Breast
Feature extraction
Tumor growth modeling