|
ACCESS THE FULL ARTICLE
No SPIE Account? Create one
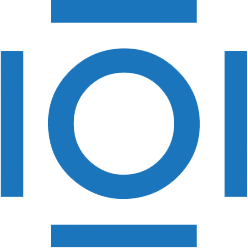
CITATIONS
Cited by 2 scholarly publications.
Classification systems
Data fusion
Neural networks
Error analysis
Mining
Wavelets
Feature extraction