|
ACCESS THE FULL ARTICLE
No SPIE Account? Create one
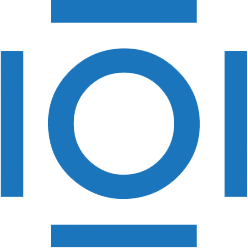
CITATIONS
Cited by 69 scholarly publications and 1 patent.
Feature selection
Principal component analysis
Proteins
Independent component analysis
Luminescence
Neural networks
Fractal analysis