|
ACCESS THE FULL ARTICLE
No SPIE Account? Create one
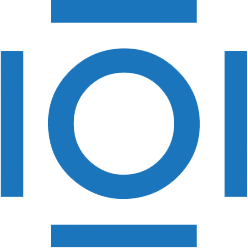
CITATIONS
Cited by 36 scholarly publications.
Visualization
Inspection
Associative arrays
Principal component analysis
Optical inspection
Data mining
Defect detection