|
ACCESS THE FULL ARTICLE
No SPIE Account? Create one
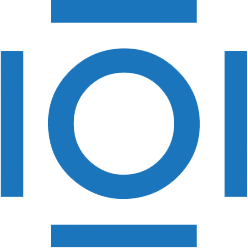
CITATIONS
Cited by 1 scholarly publication.
Wavelets
Neural networks
Damage detection
Wavelet transforms
Digital filtering
Fourier transforms
Artificial neural networks