|
ACCESS THE FULL ARTICLE
No SPIE Account? Create one
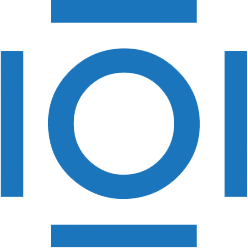
CITATIONS
Cited by 2 scholarly publications.
Complex systems
Structural dynamics
Systems modeling
Computing systems
Aerospace engineering
Motion models
Dynamical systems