|
ACCESS THE FULL ARTICLE
No SPIE Account? Create one
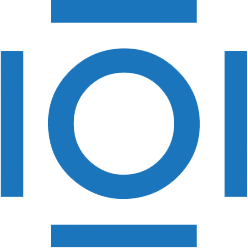
CITATIONS
Cited by 2 scholarly publications.
Brain
Functional magnetic resonance imaging
Brain mapping
Statistical analysis
Neuroimaging
Signal processing
Image resolution