|
ACCESS THE FULL ARTICLE
No SPIE Account? Create one
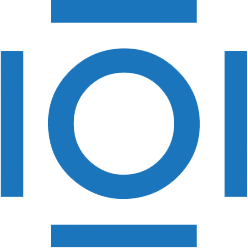
CITATIONS
Cited by 2 scholarly publications.
Principal component analysis
Clouds
Lithium
Reconstruction algorithms
Computer graphics
Data modeling
Computer science