|
ACCESS THE FULL ARTICLE
No SPIE Account? Create one
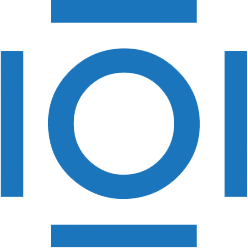
CITATIONS
Cited by 5 scholarly publications.
Wavelets
Wavelet transforms
Structural health monitoring
Monte Carlo methods
Wave propagation
Error analysis
Signal processing