|
ACCESS THE FULL ARTICLE
No SPIE Account? Create one
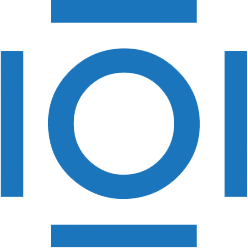
CITATIONS
Cited by 1 scholarly publication.
Lawrencium
Image segmentation
Image classification
Image processing algorithms and systems
Radon
Hyperspectral imaging
Expectation maximization algorithms