|
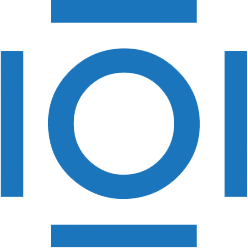
CITATIONS
Cited by 35 scholarly publications.
Image segmentation
Remote sensing
LIDAR
Neural networks
Image fusion
Image classification
RGB color model