Accurate information on the spatial distribution of crops is of great significance for scientific research and production practices. Such accurate information can be extracted from high-spatial-resolution optical remote sensing images. However, acquiring these images with a wide coverage is difficult. We established a model named multispectral super-resolution generative adversarial network (MS_SRGAN) for generating high-resolution 4-m images using Gaofen 1 wide-field-view (WFV) 16-m images. The MS_SRGAN model contains a generator and a discriminator. The generator network is composed of feature extraction units and feature fusion units with a symmetric structure, and the attention mechanism is introduced to constrain the spectral value of the feature map during feature extraction. The generator loss introduces feature loss to describe the feature difference of the image. This is realized using pre-trained discriminator parameters and a partial discriminator network. In addition to realizing feature loss, the discriminator network, which is a simple convolutional neural network, also realizes adversarial loss. Adversarial loss can provide some fake high frequency details to the generator to get a more sharpened image. In the Gaofen 1 WFV image test, the performance of MS_SRGAN was compared with that of Bicubic, EDSR, SRGAN, and ESRGAN. The results show that the spectral angle mapper (3.387) and structural similarity index measure (0.998) of MS_SRGAN are higher than those of the other models. In addition, the image obtained by MS_SRGAN is more realistic; its texture details and color distribution are closer to the reference image to a greater extent. |
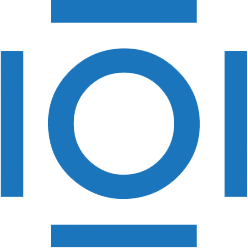
CITATIONS
Cited by 6 scholarly publications.
Super resolution
Remote sensing
Feature extraction
Image fusion
Convolution
Data modeling
Multispectral imaging