Urban forestry is regarded as the green infrastructure of cities, the analysis of which is of great significance for research on statistical analysis, such as greening, carbon sink, etc. on the basis of remote sensing detection. However, current urban forestry detection algorithms are focused on typical machine learning, whose results are limited by the manually selected features. Therefore, an innovative detection algorithm for analyzing urban forestry is proposed. First, the simple linear iterative clustering algorithm is adopted to segment the dataset, and the edge information of the object is preserved as much as possible. Second, a new network structure is constructed on the basis of Mask-RCNN to refine the detection results: (1) dilated convolution is adopted to replace the normal convolution of C1 in backbone (ResNet101) to increase the size of receptive field; (2) convolutional block attention module is introduced in C2 to give more weight to the shallow features such as edges and colors; and (3) atrous spatial pyramid pooling (ASPP) is added to branch of C3, enhancing the ability of multiscale fusion. With the effect of ASPP on detection results at different positions of backbone compared, the results show that the more backward the ASPP is, the more obvious the trend of negative correlation between network convergence speed and detection accuracy (C3: 89.2%, C4: 84.3%, and C5: 81.6%) is. The accuracy of random forest and support vector machines is also compared, with the former of 69.4% and the latter of 71.2%. In addition, the method of transfer learning to unmanned aerial vehicle data is used in this research, with the accuracy of 81.6%. The result shows that the improved segmentation model features better ability of learning shallow features than the original, able to accurately extract urban forestry from remote sensing images, which provides the reference for the further research on urban forestry detection, mapping, as well as the multisource remote sensing data fusion. |
ACCESS THE FULL ARTICLE
No SPIE Account? Create one
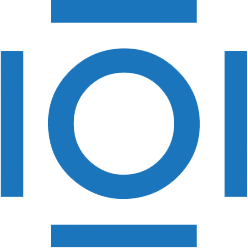
CITATIONS
Cited by 5 scholarly publications.
Image segmentation
Remote sensing
Forestry
Data modeling
Unmanned aerial vehicles
Convolution
Satellites