Significance: Accurate early diagnosis of malignant skin lesions is critical in providing adequate and timely treatment; unfortunately, initial clinical evaluation of similar-looking benign and malignant skin lesions can result in missed diagnosis of malignant lesions and unnecessary biopsy of benign ones. Aim: To develop and validate a label-free and objective image-guided strategy for the clinical evaluation of suspicious pigmented skin lesions based on multispectral autofluorescence lifetime imaging (maFLIM) dermoscopy. Approach: We tested the hypothesis that maFLIM-derived autofluorescence global features can be used in machine-learning (ML) models to discriminate malignant from benign pigmented skin lesions. Clinical widefield maFLIM dermoscopy imaging of 41 benign and 19 malignant pigmented skin lesions from 30 patients were acquired prior to tissue biopsy sampling. Three different pools of global image-level maFLIM features were extracted: multispectral intensity, time-domain biexponential, and frequency-domain phasor features. The classification potential of each feature pool to discriminate benign versus malignant pigmented skin lesions was evaluated by training quadratic discriminant analysis (QDA) classification models and applying a leave-one-patient-out cross-validation strategy. Results: Classification performance estimates obtained after unbiased feature selection were as follows: 68% sensitivity and 80% specificity with the phasor feature pool, 84% sensitivity, and 71% specificity with the biexponential feature pool, and 84% sensitivity and 32% specificity with the intensity feature pool. Ensemble combinations of QDA models trained with phasor and biexponential features yielded sensitivity of 84% and specificity of 90%, outperforming all other models considered. Conclusions: Simple classification ML models based on time-resolved (biexponential and phasor) autofluorescence global features extracted from maFLIM dermoscopy images have the potential to provide objective discrimination of malignant from benign pigmented lesions. ML-assisted maFLIM dermoscopy could potentially assist with the clinical evaluation of suspicious lesions and the identification of those patients benefiting the most from biopsy examination. |
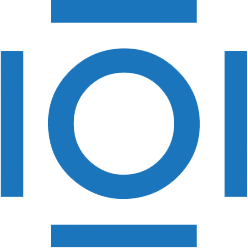
CITATIONS
Cited by 3 scholarly publications.
Skin
Melanoma
Luminescence
Tumor growth modeling
Data analysis
Skin cancer
Biopsy