|
ACCESS THE FULL ARTICLE
No SPIE Account? Create one
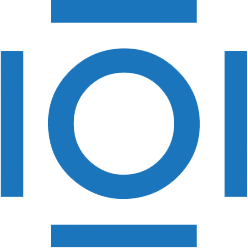
CITATIONS
Cited by 5 scholarly publications.
Wavelets
Brain
Neuroimaging
Independent component analysis
Image classification
Feature extraction
Magnetic resonance imaging