|
ACCESS THE FULL ARTICLE
No SPIE Account? Create one
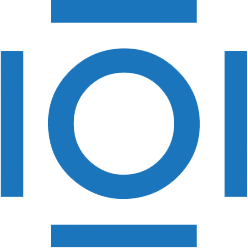
CITATIONS
Cited by 27 scholarly publications and 1 patent.
Algorithms
Image processing
Algorithm development
Expectation maximization algorithms
Databases
Chemical elements
Data mining