|
ACCESS THE FULL ARTICLE
No SPIE Account? Create one
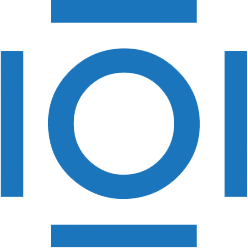
CITATIONS
Cited by 2 scholarly publications and 1 patent.
Detection and tracking algorithms
Binary data
Fourier transforms
Statistical modeling
Head
Distance measurement
Optical spheres