|
ACCESS THE FULL ARTICLE
No SPIE Account? Create one
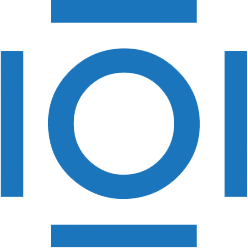
CITATIONS
Cited by 1 scholarly publication.
Roads
RGB color model
Image processing
Detection and tracking algorithms
Image segmentation
Lutetium
Video processing