|
ACCESS THE FULL ARTICLE
No SPIE Account? Create one
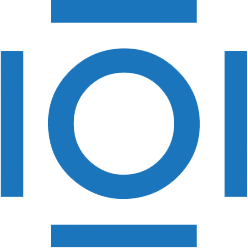
CITATIONS
Cited by 12 scholarly publications.
Binary data
Image analysis
Image resolution
Chemical elements
Data modeling
Model-based design
Image retrieval