Free-hand sketches play a vital role in graphically portraying ideas and concepts in image recognition systems. Most recently proposed learning-based sketch recognition methods have achieved marked progress in recognition accuracy, but they rarely optimize the use of the sparsity features of sketch images. Although several attention-based sketch recognition models have been presented, they endure complex computations and large model sizes. To address these challenges, we present a lightweight convolutional neural network called Light-SRNet based on a dual-attention mechanism to improve the accuracy of sketch recognition while retaining its lightweight nature. In the proposed model, we introduced both the spatial and channel attention mechanisms into the feature extraction network to highlight more discriminative feature representations to enhance its powerful sketch recognition ability. We compared the proposed Light-SRNet with its competitors on the TU-Berlin dataset, Sketchy dataset, and QuickDrawExtended dataset. Extensive experimental results show that Light-SRNet achieves a recognition accuracy of 73.14%, which is comparable to other similar sketch recognition techniques, while requiring only about a quarter of the model parameters. |
ACCESS THE FULL ARTICLE
No SPIE Account? Create one
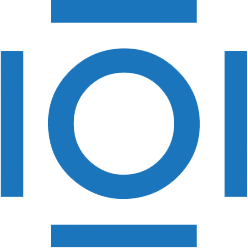
CITATIONS
Cited by 1 scholarly publication.
Feature extraction
Education and training
Performance modeling
Data modeling
Feature fusion
Convolution
Visualization