Metal gaskets are an essential component of the manufacturing industry, and detecting end-face defects is critical to ensuring their quality. However, current detection methods struggle to detect such defects due to the gaskets’ uneven end-face structure and small defect size. To address such issues, an approach called SR-HorNetYOLO has been proposed. This approach combines traditional machine vision and deep learning techniques. The visual saliency method is used to simplify the image and remove irrelevant features. YOLOv5 is then optimized in three ways: the GhostNet backbone replaces the CSPDarkNet53 backbone to reduce model weight, the self-attention mechanism and bottleneck transformer block improve the precision of detecting small targets, and the feature pyramid is improved through the HorNet network to enhance the predictive performance of the network. Finally, a classification module and position module are employed to classify and locate three-fusion proportion characteristic images. The experimental results show that the SR-HorNetYOLO method achieves a precision of 93.3% and a recall rate of 94.0%. |
ACCESS THE FULL ARTICLE
No SPIE Account? Create one
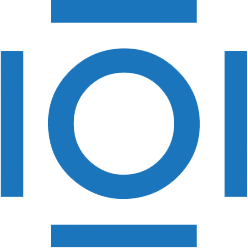
CITATIONS
Cited by 1 scholarly publication.
Defect detection
Metals
Detection and tracking algorithms
Convolution
Feature extraction
Image processing
Object detection