|
ACCESS THE FULL ARTICLE
No SPIE Account? Create one
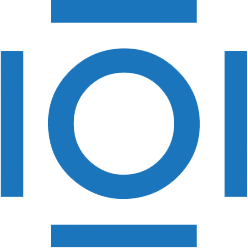
CITATIONS
Cited by 1 scholarly publication.
Image segmentation
3D modeling
Magnetic resonance imaging
3D image processing
Algorithm development
Computed tomography
Barium