Purpose: Accurate classification of COVID-19 in chest radiographs is invaluable to hard-hit pandemic hot spots. Transfer learning techniques for images using well-known convolutional neural networks show promise in addressing this problem. These methods can significantly benefit from supplemental training on similar conditions, considering that there currently exists no widely available chest x-ray dataset on COVID-19. We evaluate whether targeted pretraining for similar tasks in radiography labeling improves classification performance in a sample radiograph dataset containing COVID-19 cases. Approach: We train a DenseNet121 to classify chest radiographs through six training schemes. Each training scheme is designed to incorporate cases from established datasets for general findings in chest radiography (CXR) and pneumonia, with a control scheme with no pretraining. The resulting six permutations are then trained and evaluated on a dataset of 1060 radiographs collected from 475 patients after March 2020, containing 801 images of laboratory-confirmed COVID-19 cases. Results: Sequential training phases yielded substantial improvement in classification accuracy compared to a baseline of standard transfer learning with ImageNet parameters. The test set area under the receiver operating characteristic curve for COVID-19 classification improved from 0.757 in the control to 0.857 for the optimal training scheme in the available images. Conclusions: We achieve COVID-19 classification accuracies comparable to previous benchmarks of pneumonia classification. Deliberate sequential training, rather than pooling datasets, is critical in training effective COVID-19 classifiers within the limitations of early datasets. These findings bring clinical-grade classification through CXR within reach for more regions impacted by COVID-19. |
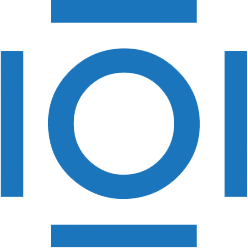
CITATIONS
Cited by 2 scholarly publications.
COVID 19
Education and training
Chest imaging
Radiography
Deep learning
Image classification
Performance modeling