Purpose: Radiologists exhibit wide inter-reader variability in diagnostic performance. This work aimed to compare different feature sets to predict if a radiologist could detect a specific liver metastasis in contrast-enhanced computed tomography (CT) images and to evaluate possible improvements in individualizing models to specific radiologists. Approach: Abdominal CT images from 102 patients, including 124 liver metastases in 51 patients were reconstructed at five different kernels/doses using projection domain noise insertion to yield 510 image sets. Ten abdominal radiologists marked suspected metastases in all image sets. Potentially salient features predicting metastasis detection were identified in three ways: (i) logistic regression based on human annotations (semantic), (ii) random forests based on radiologic features (radiomic), and (iii) inductive derivation using convolutional neural networks (CNN). For all three approaches, generalized models were trained using metastases that were detected by at least two radiologists. Conversely, individualized models were trained using each radiologist’s markings to predict reader-specific metastases detection. Results: In fivefold cross-validation, both individualized and generalized CNN models achieved higher area under the receiver operating characteristic curves (AUCs) than semantic and radiomic models in predicting reader-specific metastases detection ability (p < 0.001). The individualized CNN with an AUC of mean (SD) 0.85(0.04) outperformed the generalized one [AUC = 0.78 ( 0.06 ) , p = 0.004]. The individualized semantic [AUC = 0.70 ( 0.05 ) ] and radiomic models [AUC = 0.68 ( 0.06 ) ] outperformed the respective generalized versions [semantic AUC = 0.66 ( 0.03 ) , p = 0.009; radiomic AUC = 0.64 ( 0.06 ) , p = 0.03]. Conclusions: Individualized models slightly outperformed generalized models for all three feature sets. Inductive CNNs were better at predicting metastases detection than semantic or radiomic features. Generalized models have implementation advantages when individualized data are unavailable. |
ACCESS THE FULL ARTICLE
No SPIE Account? Create one
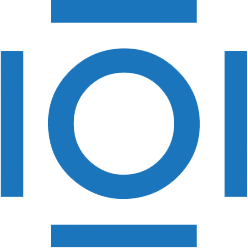
CITATIONS
Cited by 1 scholarly publication.
Performance modeling
Liver
Computed tomography
Feature extraction
Data modeling
3D modeling
Radiology