|
ACCESS THE FULL ARTICLE
No SPIE Account? Create one
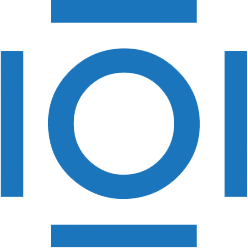
CITATIONS
Cited by 2 scholarly publications.
Classification systems
Reliability
Image classification
Optical engineering
Sun
Information fusion
Binary data