We present an accurate and efficient transformation estimation algorithm called local reference frame-based maximum consensus statistic (LRF-MCS). Moreover, a local reference frame (LRF) is proposed for effectively implementing our LRF-MCS. The proposed LRF uses a small local region to calculate its z axis and employs a salient local point to determine its x axis for improving the robustness to incomplete region. The proposed LRF-MCS first efficiently calculates the consensus set connected to each input correspondence by an LRF-based transformation technique. Then a switch program is used to select an appropriate consensus set for calculating the optimal transformation, which ensures the strong robustness to the seriously corrupted input correspondences. Extensive experiments are implemented on four standard datasets for comprehensively evaluating the performance of the proposed LRF and LRF-MCS techniques. The experimental results show that our LRF and LRF-MCS techniques outperform the state-of-the-art algorithms in terms of accuracy, efficiency, and robustness, achieving the best overall performance. |
ACCESS THE FULL ARTICLE
No SPIE Account? Create one
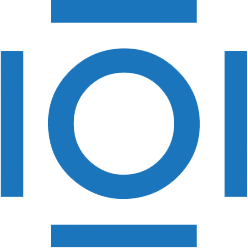
CITATIONS
Cited by 1 scholarly publication.
Laser range finders
3D modeling
Clouds
Optical engineering
Statistical analysis
Error analysis
Image registration