|
ACCESS THE FULL ARTICLE
No SPIE Account? Create one
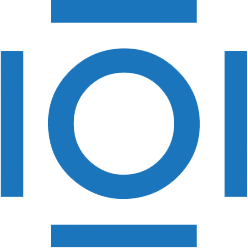
CITATIONS
Cited by 2 scholarly publications.
Detection and tracking algorithms
Edge detection
Computer vision technology
Machine vision
Feature extraction
Resistance
Signal processing