|
ACCESS THE FULL ARTICLE
No SPIE Account? Create one
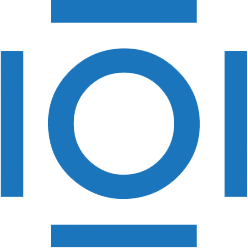
CITATIONS
Cited by 7 scholarly publications and 3 patents.
Photomasks
Inspection
Defect inspection
Lithography
Image classification
SRAF
Defect detection