|
ACCESS THE FULL ARTICLE
No SPIE Account? Create one
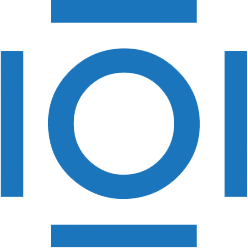
CITATIONS
Cited by 3 scholarly publications and 4 patents.
Neural networks
Diffusion
Neurons
Data modeling
Optical proximity correction
Photoresist processing
Finite element methods