1.IntroductionThis study realizes a further step in a series of publications to reveal, and help limit, the contribution of mask defectivity in the stochastics of extreme ultraviolet (EUV) imaging. Previous studies1,2 showed this for local defects and several types of non-local mask deficiencies (NLMDs). These were first simulated for simple line-and-space [i.e., one-dimensional (1D)] patterns emulating the 0.33-NA EUV scanner used in high volume manufacturing. Later works3,4 expanded this to imaging at 0.55 NA, accounting for the anamorphism as specified in ASML’s EXE5000 system, and made comparisons between imaging at either NA, in view of stochastics and possible differences between horizontal and vertical direction imaging for 0.55 NA. Gradually, mask roughness became the most studied type of NLMD. Our preceding publication5 was the first to suggest confinement of mask roughness for 0.55 NA. This was based on a 1D study, with only an outlook toward two-dimensional (2D) patterning included. This extension to 2D 0.55-NA is the intent of the present paper. This study examines an emulated metal interconnect pattern. The 2D pattern is a staggered placement of 60-nm long, 11-nm wide trenches (2D spaces) at a 22-nm pitch with a target tip-to-tip gap (T2T) at 16 nm of photoresist. The horizontal pattern is sketched in Fig. 1. The vertical pattern follows the same specifications but is rotated by 90 deg. Fig. 1Horizontal 2D pattern used in this study. It is intended to emulate a metal interconnect layer while challenging lithography. The same pattern rotated 90 deg is examined in the vertical direction. ![]() With all further work undertaken at 0.55 NA, Sec. 2 first details our simulation setup, expanding it for the selected 2D pattern. Section 3 then presents results based on brute force use of stochastic simulations. Next, Sec. 4 addresses the previously suggested alternative roughness threshold technique based on background intensity in the pupilgram.5 Finally, Sec. 5 discusses the results and draws conclusions on what insights this adds to mask roughness contribution for imaging of arbitrary 2D patterns. It is worth noting that all values in this manuscript are presented at the wafer scale unless specifically designated otherwise. 2.Simulation SetupThe data for this study were generated from stochastic computational simulations. The simulation platform is Sentaurus Lithography EUV 2021.12. (S-Litho) with Process Window Analyzer 2021.12 from Synopsys Inc and Python scripts to work with the output stochastic data. The S-Litho simulations were performed with a waveguide algorithm.6,7 Optical proximity correction was performed using Proteus 2021.12 from Synopsys Inc. 2.1.Lithography SimulationThe mask, resist, and scanner values are expected to be relevant for manufacturing on a 0.55-NA EUV lithography system under development. 2.1.1.Scanner simulationThis study emulates the projected EXE:5000 0.55 NA EUV scanner from ASML.8 Table 1 contains specific scanner settings applied on the simulations. Table 1Simulation parameters used in studies.
Note: Mask material thickness input parameters are defined at mask scale (MS), and appropriate remaining parameters are defined at wafer scale. The illumination is aligned to the primary pattern direction using leaves as found in Fig. 2. The leaf sizes and placements are set for an 11-nm line and 11-nm space pattern. The same illumination is used for both 1D and 2D patterns. The obscuration is a circular approximation given by the scanner manufacturer for use during development and publication.9 Fig. 2Scanner optical system configuration in wafer angular coordinates. Horizontal and vertical leaf illuminators are used for horizontal and vertical orientated patterns, respectively. The horizontal small angle pole is the top leaf and the large angle pole is the bottom leaf. The projection system uses by magnification with 0.55 NA and a central obscuration. Table 1 lists the numerical inputs. ![]() 2.1.2.Resist simulationTo study stochastic defects in simulation, it is necessary to increase the defectivity ratio of the modeled system such that defects are detected at computationally efficient rates in the limited simulation area.11 The resist model was modified to increase defectivity to a rate of for a simulated area of . This modification is named the Suppressed EUV CAR model and is incorporated from previous work.4 A stochastic failure window (SFW) for the suppressed EUV model is shown in Fig. 3, as calculated on a substrate with a simulated multilayer ripple (MLR.) The MLR increased defectivity for the 11-nm target line CD to above the 2:20,000 unperturbed target. Parameters for the suppressed EUV CAR model are presented in Appendix 1. Fig. 3Simulated SFW for a perturbed suppressed EUV CAR resist line CD measurements. The simulated data were collected on a 6.25-pm rms 5-nm (correlation length, discussed in Sec. 2.1.3) ripple substrate. ![]() The photoresist is applied on an underlayer, and the underlayer is on a silicon substrate. Optical parameters for these materials are found in Appendix 2. 2.1.3.Photomask simulationWafer scale photomask parameters are given in Table 1. The EUV mask is perturbed in this study through various MLR rms and correlation length () variations. Printed wafer defectivity is simulated and studied stochastically for each MLR variation combination. MLR approximates mask low thermal expansion material (LTEM) and multilayer reflector (ML) variations for stochastic study. The perturbance range in the direction applied at the ML LTEM interface ranges from 6.25 to 12.5 pm. MLR is applied at the wafer scale and projected through the anamorphic optics. The MLR is randomly generated using a random seed, a root mean square amplitude (rms) in nanometers, and a correlation length () in nanometers, as found in Eq. (1). The MLR function is calculated for a region that is normally sized from and in nanometers at the wafer scale and on a grid sized (nm). The rms value defines the variation magnitude into the ML or direction. The function blends each randomly generated rms value together into a smoother surface. RAND is a random number generator that generates a random number from to 1 using a seed, , and The first ML is conformally mapped to the rippled LTEM, and each subsequent ML is conformally mapped to the ML below it. This distortion propagates into the ML cap and the absorber as apparent in Fig. 4.Fig. 4Simulated MLR generation in an EUV mask. (a) The mask cross section shows randomly generated LTEM substrate roughness at the ML stack bottom. The simulated ripple produces waves in the ML layers (referred to as ripple in these studies) and the absorber. The three dimensional and 2D plots map the same randomly generated ripple shape applied to the LTEM, respectively, (b) in perspective view and in (c) top-view as a contour plot. ![]() To reduce computation time, simulate regions are made periodic whenever possible. In this study, the tests patterns are periodic in the and directions. However, MLR is not periodic. This introduces error around the simulation region edge. The error is considered acceptable due to the runtime savings and the amount of error, which is on the order of 0.1 to 0.3 nm of CD for an 11-nm feature when the feature is within of the edge. To reduce the error’s impact, measurements are not calculated within 20 nm of the simulation edge. The applied MLR map is larger than the simulation area. Figure 5 shows how horizontal and vertical versions of the same pattern are placed with respect to the MLR. The simulation procedure attempts to use the same MLR map region for all simulations whenever possible. However, an exact overlap is not always possible as in the case of the horizontal and vertical 2D structure simulation areas outlined in Fig. 5. Also, even when MLR mapped regions overlap, the measurement locations are not coincident due to the rectangular simulation shape precluding exact MLR comparisons for horizontal and vertical cases. Fig. 5Applied portion of the substrate map, for an example ML-ripple, respectively, for horizontal (red) and vertical (white) orientation of lines-and-spaces patterns. The primary measurement regions overlap, but the exact T2T and trench CD measurement locations are offset from each other due to the shape of the simulation area as seen in the right image. This is more pronounced the further the measurement point is from the center of the defined MLR region, which is the rotation point. ![]() 2.2.Optical Proximity Correction1D features were imaged by adjusting dose to achieve the desired space width in photoresist on the wafer. 2D features required optical proximity correction to achieve target resist shapes. A simple optical proximity correction (OPC) was performed using the continuum (non stochastic) version of the model. Correction engine response to stochastic input will result in an OPC targeted for a specific stochastic case, if OPC is even able to achieve a convergent stochastic solution. During OPC, the T2T on wafer was targeted at 16 nm, and it was the primary driving factor for OPC. The wafer trench width of 11 nm was a secondary correction consideration. The short nature of the trenches (60 nm) and the proximity of large numbers of corners to every point on the trench edges led to significant proximity effects. A more complex correction for the T2T CD and trench CD is necessary to fully suppress these effects and maintain tight CD control, unlike the simple correction used in this study. Figure 6 shows the corrected patterns that were generated for this simulation. Independent corrections were made for the horizontal and vertical patterns with a unique model and correction set for each orientation. The leaf orientation requires a unique correction model for the two orientations. The orientation-specific correction produces tighter corrected T2T gaps in the vertical trench orientation (between top and bottom of the T2T) on the mask than in the horizontal orientation (between the upper to lower edges of the trench). The corrected horizontal trench correction is asymmetric. Fig. 6OPC applied to horizontal and vertical 2D patterns on the dark field reticle. The gray region is the absorber, and the red shapes are the Ru ML stack cap. The orange lines indicate locations where wafer trench CDs are simulated. ![]() Simulation time of the 2D pattern is considerably greater than simulation time for the 1D patterns. The 1D pattern used in previous work4,11 is 66 by 60 nm, and the 2D pattern is 148 by 88 nm for both horizontal and vertical configured patterns. This constitutes an approximately 325% area increase for the 2D pattern simulation. Using the same hardware and software configuration, the 2D pattern runtime is longer than the 1D simulation. The increase in runtime is primarily due to the increase in area but also to some algorithmic advantages found in the uniform 1D patterns. The runtime reduced the simulation runs available for the 2D study. 2.3.Stochastic Simulation MethodologyEach stochastic data point analyzed in this study is normally derived from 20,000 simulations. The simulated points are obtained through of computation on 20 CPUs for 1D patterns. This time and computation resource consumption was considered acceptable for data generation in this study. Outside of the suppressed resist model and S-litho computational efficiencies, the stochastic results were numerically calculated in a brute force manner. The simulation uses pseudo random numbers to model stochastic effects. A pseudo random number uses a seed in a pseudo random number generator function to produce a random value as found in Eq. (1). Every time a given seed is used in a pseudo random number generator, the same random value is produced. The Mersenne Twister12 is used to generate random values in this study. A pseudo random number has an advantage in a simulated stochastic study in that it allows the investigator to reproduce any stochastic case on demand by rerunning with the same seed. The random seed number is used to name a particular run or element in a stochastic study of this nature. Each trial in the 20,000 trials shown in Fig. 7 consisted of the seven stochastic elements listed in Table 2. These stochastic terms are the initial post exposure bake (PEB) conditions and interaction and kinetic descriptions of the stochastic PEB process. In this study, these seven random terms always used the same random seed. Although each component used the same seed, each term generated its own independent random values from that seed. Fig. 7Example of a stochastic run with 20,000 points (1D pattern) and simulated bridging failures. The horizontal axis is the random seed [seed in Eq. (1)] used to generate the pseudo random number for a data point, and the vertical axis is the simulated trench CD for each point. The target CD is 11 nm. The points marked as bridging failures measure at times trench CD, which is the result of a trench filled with photoresist and the two lines adjacent to the filled trench (.) The large CD range is due to the use of the defect generating suppressed EUV resist model. ![]() Table 2Stochastic terms used in simulation.
When certain combinations of the seven random seeds arise, wafer level resist feature failures are observed. In Fig. 7, eight of the 20,000 test cases produce trench bridging that produces a failing trench feature. This is because only 1:2500 times in the Fig. 7 case did the generated stochastic values combine to produce a failure as in the right image in Fig. 7. It is worth noting that the observed failure ratio and CD variation in Fig. 7 and all other plots in this study are orders of magnitude higher than that observed in the experiment due to the use of the suppressed resist model. This is due to the need to produce stochastic failures in a reasonable computation time to study stochastic failures. 2.4.Simulation Data AnalysisA simulated stochastic CD is generated for each location indicated in Fig. 6. These CDs are analyzed together to generate data points found later in this study. Most data points presented in this study are the statistical combination of 20,000 stochastic trials. The total number of points () in a trial such as Fig. 7 is comprised of the number of good points () and the number of failed points () per Eq. (2): A good point () must meet two criteria. First, must produce a measurement value. Points that do not produce measurement values either have no simulated CD resulting from defective resist patterns such as a resist filled trench or a missing line. The second criterion is that must fall within a range of CDs that are useful for analysis. The criteria are feature dependent. This range is set much wider than the range that is traditionally found in manufacturing. This increased range facilitates the study of distributions in a wider range of conditions. In addition, the trench and T2T criteria use different values. The variable is the individual trial being checked against the criteria for a feature. Equation (3) describes the criteria for trenches: The T2T maximum criteria are based on the analysis in Fig. 8, where the T2T gap and half of the rounded up via size are the failure limit. This is a potential half via coverage that could lead to an open in a final product. Equation (4) holds the definition for T2T features using these criteria: Fig. 8T2T failure specification. The purple squares are 11 nm by 11 nm vias on a corresponding via layer, and the T2T gap in the metal layer is 16 nm. The maximum failure spec is the T2T gap and half the 11 nm via size, rounded up to an integer value of 22 nm. for the T2T gap is and . ![]() A failed () point is any point in the simulation set that is not a good point per Eq. (5): Statistics are calculated using only the values and . Equations (6) and (7) describe the data included in average and standard deviation calculations, respectively, The failure ratio is described in Eq. (8): 3.Simulation OutputSimulation data were generated and analyzed per Sec. 2 and are presented here. 2D feature data were generated for this study, and some one-dimensional data are included from previous work for comparison, as noted. 3.1.Two-dimensional Feature Defectivity2D pattern features (2D features) are features that are optically impacted in both the horizontal and vertical directions. Corners such as line-ends and jogs are readily identified as 2D features. However, trench edges may also be 2D in nature even when they are part of a straight edge. Figures 6 and 9 show the corrected and uncorrected 2D pattern used in this study. Every point on every edge of each polygon in Fig. 9 is or less from a corner. This indicates that every point is influenced by a corner, and therefore every point is 2D in nature. This indicates that all edges in Fig. 9 will experience proximity effects that require damping during OPC. Fig. 92D feature failure ratios by the wafer CD, feature type, and . The layout depicts the measurement location for each feature in the plot: green horizontal dashed oval—T2T; maroon vertical solid oval—trench CI; and blue vertical dotted oval—trench DI. Each feature is plotted with four simulated values in nm at the wafer scale. Each point results from 20,000 simulations. ![]() The plot in Fig. 9 contains trenches and T2T structures. The trenches are split into two groups based on their measurement locations on the trench. The constructive interference (CI) group is placed at the first standing wave node along the trench edge from the line-end. The standing waves produce a bulge in the wafer contour at the CI measurement location as seen in the resist plot in Fig. 10. The destructive interference (DI) location is the second standing wave location from the trench’s end along its edge. A constriction is produced in the wafer contour at the DI location and the OPC bulges the mask pattern out around the DI location as found in the layout in Fig. 10. Further enhancing the OPC correction and improving dose targeting should make Fig. 9 constructive and DI defectivity trends colinear. Fig. 10(a) The constructive and DI measurement locations on a wafer stochastic photoresist trench simulation and (b) the corresponding OPC. The constructive interference locations circled in solid maroon tend to bulge in resist compared with as-drawn, whereas the DI locations tend to pinch in resist compared with as-drawn. The OPC treatment (b) dramatically reduces or even eliminates the effect for some of the locations. The stochastic nature of the simulation produces the varied response across the pattern. The elevated defectivity in the applied resist model tends to exaggerate the interference behavior in the photoresist. This is a desired effect, as it generates observable defects in simulation with increased probability. ![]() The T2T and trench features appear to follow a consistent, yet noisy, trend between wafer CD and failure ratio. The three feature classes are subdivided into groupings as indicated by plot markers in Fig. 9. However, detailed analysis of Fig. 9 finds no significant correlation between and the failure ratio, except for the 0 case. 3.2.1D and 2D Horizontal and Vertical Feature DefectivityThe orientation based defectivity performance of 1D and 2D features in a 0.55-NA EUV system is important, especially with the anamorphic nature of the system. Horizontal features are drawn parallel to the slit direction and perpendicular to the scan direction and chief ray angle, whereas vertical features are perpendicular to horizontal features as shown in Fig. 11. Vertical trenches are drawn in the magnification direction, with upper and lower trench-ends subjected to shadowing effects. Horizontal trenches are drawn in the magnification direction, and their width is subjected to shadowing. Fig. 11Horizontal and vertical 1D and 2D patterns. 1D patterns are shown in black in the top row, and 2D patterns are in red in the bottom row. The horizontal pattern direction is parallel to the slit direction, and the vertical pattern direction is parallel to the scan direction. ![]() As found in Fig. 12, trenches in a 1D pattern demonstrate a significantly lower failure ratio when imaged horizontally than when imaged vertically. Each horizontal space generates an lower failure ratio for any wafer space CD than the corresponding vertical space CD. This phenomenon remains consistent across all of the stochastically generated CD values where both horizontal and vertical pattern values are present. This is interpreted due to the larger magnification in the scan direction. Fig. 12Failure ratio for horizontal and vertical one dimensional 11 nm trenches by the wafer CD. The trenches are simulated at best focus using a 12.5-pm rms and 20-nm MLR. The horizontal trenches consistently demonstrate a lower failure ratio than the vertical features. Each point is derived from 20,000 simulations. ![]() Figure 13 plots the T2T failure ratios for horizontal and vertical features. The 2D simulations require significantly longer simulation times than the 1D features due to the required simulation area. This increased simulation time resulted in fewer 2D simulation runs; therefore 3 defocus conditions are included in Fig. 13. The included defocus values of – 5, 0, and 5 nm produced no detectable defect variation trend with respect to defocus. The defocus values have more stochastic data points, which increases the quantity of data presented and thus increases the stochastic variation observed between the simulated data points found in Fig. 13. Fig. 13Failure ratio for horizontal and vertical 16-nm T2T gaps by the wafer CD. These are simulated at a defocus of −5, 0, and 5 nm, in the case of a 12.5-pm rms and 20-nm MLR. The vertical orientation generally outperforms the horizontal one. All points are derived from 20,000 simulations. ![]() The wafer defectivity versus orientation trend is reversed in Fig. 13 compared with Fig. 12. In Fig. 13, the vertical T2T features generally have lower wafer defectivity below 16 nm. Above that, they have similar wafer defectivity levels. These data are significantly noisier than the 1D case, making a direct comparison difficult, especially for values greater than 16 nm. Part of the reason for the noise is the low number of defects found in both the vertical and horizontal cases with many points representing only 0 to 5 defects – the simulated defects found below in Fig. 13. These defect counts mean that the 20,000 simulation steps are not finding many defects, so the confidence in the defect count is lower. Confidence refers to the probability of a similar defect value occurring in a subsequent simulation. The number of steps could be increased to better understand defectivity trends and increase confidence. The vertical case appears to have a wider distribution than the horizontal case in Fig. 13. However, it is important to note that the vertical case reaches the simulated defectivity floor where no defects are found and it has many points with 1:20,000 or 2:20,000 failures (). The floor spreads the vertical points below 16 nm. The horizontal case never reaches the defectivity floor with a best defectivity of 2:20,000. This creates a defect spread in the low defect region that gives the appearance of increased noise in the vertical orientation versus the horizontal orientation. 3.3.Multilayer Ripple Stochastic Wafer Defectivity ImpactMultilayer ripple influence on wafer features is a function of and rms. Spaces (trenches) in 1D feature configuration were simulated with 10 random cases for an – rms configuration and measured in nine places. In 2D feature simulations, five random – rms configuration cases were used and five T2T measurements were produced from each case, as found in Fig. 6. Figure 14 shows plots of MLR values under various conditions. For each point, a random MLR with given and rms is generated. With the generated MLR, 20,000 simulations are computed using the seven random variables from Table 2 to vary the resist and secondary electrons in each simulation. The mean value of the 20,000 values determines the CD [Eq. (6)], the three sigma value is calculated using Eq. (7), and the failure ratio is the ratio of failures in the 20,000 experiments and the total number of experiments [Eq. (8)]. Fig. 14Multilayer ripple contribution to wafer stochastic defectivity for 1D and 2D features. The green points are variation (nm) of the CD plotted against the simulated average CD (nm). The magenta points are the failure ratio plotted (unitless) against the simulated average CD (nm). The No MLR case (left column) reveals the underlying stochastic variation due to the resist and secondary electron components. The 1D patterns (upper two rows) are targeted to 11-nm spaces, and the 2D patterns (bottom row) are targeted to 16-nm T2T gaps (photoresist.) Each green point is paired with a magenta point and each pair of points was generated from 20,000 simulated trails. The rms and values are shown at . The first two rows are adopted from previous work.5 ![]() The rows in Fig. 14 each contain a no MLR case in the left column. These cases illustrate the impact of the stochastic simulation components from Table 2 on the features simulated in this study, for a perfect mask. Notably, the 1D space feature demonstrates a minimal deviation in failure ratio and 3σ variation to these elements across nine measurement sites. The 2D T2T feature displays an elevated failure ratio and an increased average CD variation across all 5 measurement sites compared with the 1D feature. The top row in Fig. 14 simulates a 1D space pattern response to at a fixed 12.5-pm rms. As the is increased, the variation of the average CD around the target increases to . Both the CD variation and the failure ratio increase as the average CD decreases below the target CD, whereas both metrics improve as the average CD increases above the target CD. In the second row of Fig. 14 the MLR is fixed at 20 nm and rms is varied. The rms row follows the same trends observed in the row of Fig. 14 with the only apparent difference being in the average CD variation range. 2D T2T features are displayed in the bottom row of Fig. 14 with a 12.5-pm rms and varied . Both the 5- and 10-nm cases show an average T2T gap spread that is similar, with similar and failure ratios. The 20-nm case appears to have a larger average T2T gap spread and increased three-sigma and failure ratios compared with the 10- and 5-nm cases, although this increase is primarily driven by one point. The sample size may not be adequate to properly judge the three cases as significantly different. 4.Roughness ThresholdThe roughness threshold () is a concept introduced in previous work,5 in which it was referred to as the acceptable background intensity level in the pupilgram. It conceptually enabled rapid analysis of an MLR case (i.e., with a given combination of rms and ) to understand potential wafer defectivity. The methodology was initially introduced during the investigation of line space patterns. An overview is presented here to aid the discussion of the need for analysis application to 2D features. Amplitude in this document denotes the magnitude of light intensity. 4.1.Roughness Threshold DevelopmentThe computation time for brute force analysis of the impact of mask deficiencies on stochastic resist systems is extensive and even more so for 2D features, as previously discussed in Sec. 2.2. To reach the resist, the mask defect image must pass through the projection optics systems from the mask; therefore it leaves a signature in the pupilgram.13 The difficulty with the pupilgram is that observing the MLR contribution is problematic when the full leaf amplitude is presented in the plot, as seen in the top row of Fig. 15. However, when the leaf amplitude is capped, the MLR contribution in the pupilgram becomes apparent, as found in the bottom row of Fig. 15. In Fig. 15, for a 1D line space pattern in the perfect mask case, all light is contained in the leaf, but when MLR is introduced, a portion of the light is scattered into the background. Fig. 15Pupilgram analysis of line space masks. The left column contains a perfect mask (no MLR), and the right column contains masks with 12.5-pm rms 20 MLR. The top row holds pupilgrams with full illumination amplitudes. In the bottom row, the illumination amplitude is capped at a relative intensity value of 0.01. The capping function makes the MLR scatter in the background apparent. ![]() The relationship between the light in the background () and the total light in the pupil () was used to generate the roughness threshold () as defined in Eq. (9): The calculation is a substantial runtime reduction versus the brute force stochastic analysis of a mask deficiency.5 To use , a threshold for mask deficiencies must be calculated. For the 1D case, the threshold was calculated at 0.015, which corresponds to a level below which the 1D CD variation is . 4.2.MLR Pupilgram Amplitude Contribution for 1D and 2D FeaturesThe data in Fig. 14 make it clear that MLR impacts wafer stochastic defectivity, CD uniformity, and CD. The analysis in Sec. 4.1 further shows that MLR scatters light in the pupil from the leaf to the background area. This scatter in the 1D features is the basis for a rapid calculation of mask roughness impact on wafer stochastics. This poses the question, Does the analysis hold for 2D patterns? The left column of Fig. 16 contains pupilgrams for horizontal configurations of the 1D and 2D patterns simulated in this study with no MLR (). The 1D pattern in this case contains no light amplitude in the background, whereas the 2D pattern has significant light amplitude in the background and reduced light amplitude in the leaves compared with the 1D pattern. Fig. 16Analysis of MLR contribution to imaging via quantitative pupilgram. The top row holds pupilgrams (amplitude by pupil position) for an 11-nm line and 11-nm space pattern (far left), and the bottom row contains pupilgrams for an OPC corrected 2D pattern with 11-nm lines, 11-nm trenches, 60-nm long trenches, and 16-nm T2T gaps. The left column of pupilgrams has no MLR (). The middle column holds pupilgrams with MLR (). The rightmost column shows the calculated difference between the no MLR and MLR pupilgrams (). The yellow circle represents the maximum addressable sigma. All pupilgrams are calculated using cells. NOTE: The column amplitude value scale is renormalized to aid contrast for visual analysis. ![]() Figure 16’s middle column of pupilgrams is for the same 1D and 2D patterns with MLR (). In the case, light is scattered from the leaves into the background by the MLR introduced into the mask. The same scatter should also occur in the 2D case, but comparing the 2D and plots visually fails to reveal any difference in amplitude between the two plots, as to indicate a MLR contribution, because the 2D pattern content appears to dominate the amplitude distribution in the pupil. To visualize the MLR contribution in both cases, the difference between the and amplitudes () were calculated. However, the light amplitude in the pupilgram cells is comprised of multiple orders, so the difference must be calculated using the rms in Eq. (10): The right column of Fig. 16 was generated from Eq. (10). Visually it is evident that the 2D pattern scatters more MLR light into the background than the 1D pattern. Table 3 quantifies the maximum and minimum scatter values, which reveals a reduction in maximum 2D leaf amplitude compared with the 1D leaf, with the background amplitude increased by a factor exceeding 3. Table 3Maximum and minimum light amplitude values found in Ad for 1D for and 2D patterns. The maximum value is found in a leaf region, and the minimum value is found in the background.
The ratios in Table 4 are calculated from Eq. (11), expressed as a percentage: where the Region is leaf or background and AllAmplitude is the leaf + background amplitudes. The 1D to difference is intensity, whereas the same difference for 2D is less than half at . It is also clear that more light is scattered into the background in the 2D case than the 1D case when MLR is added into the system.Table 4Light amplitude distribution across the pupil for the selected 1D and 2D study cases. By column, each metric sums to 100%.
5.Discussion and ConclusionsThis section summarizes the findings of this mask roughness impact simulation study. The study extends MLR impact from 1D patterns used in the earlier studies5 to an emulated metal layer 2D pattern in the present one. It was previously shown that an alternative mask deficiency analysis technique based on pupilgram light amplitude distribution allows for analysis of tolerable mask roughness, without using time consuming stochastic simulations, for the 1D case. The present study investigated this for the 2D case. 5.1.MLR and DefectivityFigures 9 and 14 show an MLR impact on stochastic wafer failure ratios. In Fig. 14, the variability due to process stochastics, presented in Table 2, is observed. In the 1D case, there is almost no CD variability from the process stochastics alone, and a CD variability range was found in the 2D case. As mask deficiencies in the form of MLR with a given and rms are added and increased, the CD range becomes significant. The failure ratio and variation vary with the obtained CD. Both metrics improve for the simulated space as CD increases beyond the 11-nm target value. However, the corresponding photoresist line width is impacted by the space width; therefore the improved space metric is not necessarily indicative of a better process condition. The same argument is true for the T2T plot although the range of tolerated variation is significantly larger than for the space. In Fig. 9, rms is held constant and is varied for each of the three feature types. Inspecting the three feature types finds the markers distributed throughout the range of CD variation and within the failure ratio trend. There is also no clustering of single values in any location, except the 0 case. The space CD distribution spreads by , whereas the T2T distribution spreads by , showing that the latter is more severely affected by a given MLR. Inspection of Fig. 14 suggests a potential trigger MLR value to instigate larger CD ranges on the wafer as well as a potential saturation value. Neither of these values are found in the plots in Fig. 14 but rather must be deduced from the observed data trends. Both the 6.25- and 9-pm rms at 20 nm MLR plots show similar delta CD ranges of and , respectively, for a 2.75-pm rms step, whereas the 3.5-pm rms step to 12.5-pm rms at 20-nm more than doubles the CD range to . The doubled 25-pm rms at the 20-nm case has a similar CD range of . The same saturation can also be observed in the 10 and 20 nm at 12.5-pm rms cases, although the smaller at 12.5-pm rms cases do not show a trigger value. The T2T plots do not show a clear MLR saturation trigger case for as the 5-nm case appears at or near full MLR saturation, as in the 1D case. The stochastic effects due to resist and optical effects appear to be more significant than those found in the 1D case. It is apparent that MLR saturation occurs between 0- and 5-nm . In conclusion, the 1D pattern in this study reaches a defect ratio and CD variation saturation point between 6.25- and 9-pm rms at 20 . In addition, the 1D patterns also reach a saturation point between 0- and 2.5-nm for 12.5-pm rms. The 2D pattern’s defect ratio and CD variation saturation point is between 0- and 5-nm for 12.5-pm rms. Due to the variation observed in the 2D 0 case, it is reasonable to infer that the 2D saturation point is also between 0 and 2.5-nm similar to the 1D case, although simulations will need to be performed to verify this inference. 5.2.Horizontal and Vertical Orientation DefectivityThe space data shown in Fig. 12 demonstrate a clear difference in horizontally and vertically oriented features with respect to defect failures. Horizontally oriented 1D space features as defined by Fig. 11 consistently demonstrate a improvement in failure ratio compared with vertically oriented 1D space features. 2D T2T gaps in Fig. 13 also show a defectivity difference between the horizontal and vertical trench orientations. The T2T gap data do not present a clearly bifurcated trend as found in the 1D case. However, the vertically oriented T2T gap features reach lower failure ratios than the horizontal features for most average CD values. In addition, only the vertical T2T features have simulation cases that reach 1:20,000 and 0:20,000 failures. In both the vertical 2D pattern and the horizontal 1D pattern, the CD measurement direction is the same, parallel to the scan direction. It appears that in an anamorphic scanner, it may be advantageous to orientate potentially mask deficient CD features vertically to reduce wafer level defectivity. This implies that the magnification in the scan direction is more advantageous than shadowing or any other 3D mask effects. 5.3.Two-Dimensional Roughness ThresholdThe calculation is a useful technique for computing the impact of roughness type mask deficiencies on 1D features while reducing the need for brute force computational analysis of stochastic systems. It is unclear if the same analysis will work for 2D systems or if it is necessary for mask deficiency characterization. Analyzing the pupilgram output, it was determined that the 2D patterns shifted less light amplitude from the leaves to the background than 1D patterns, as found in Table 4. The 1D analysis has a threshold of 1.5% of amplitude. Scaling the 2D value, this reduces to of intensity, which seems too small to allow for repeatable analysis in the 2D case. Examination of the 2D cases finds differing behavior between the 1D and 2D features such as the noise found in Fig. 13 that is less apparent in Fig. 12. However, these differences do not seem to require the use of 2D features to validate mask roughness limits beyond what is found by the 1D feature analysis. 5.4.0.55-NA Experimental MLR RecommendationAs motivated above, the detailed analysis in this paper does not suggest that statements on MLR tolerances in earlier 1D5 work require updates for 2D patterns. Generalizing, the present analysis shows that 1D evaluation remains most capable at defining tolerance limits for mask roughness as done therein. The advice remains, as a starting point, to use the same limit for any 2D pattern content. Anyway, the suggested method can potentially provide new insights into how to confine that for a specific 2D pattern content or for any hotspots found in each layout. This study seems to indicate best learning is to maintain the statement that the 12.5 pm MLR roughness tolerance limits derived for 1D,5 (assumed state-of-the art) is most likely too large for 0.55-NA EUV imaging. This tentative limit is considered a good target for experimental verification work for mask roughness contribution to imaging, based on any production-capable, real-life resist process or a matched simulation model. 6.Appendix 1: Suppressed EUV CAR Resist ModelDetails of the suppressed EUV CAR model were published in previous work.4 The model parameters and their associated settings are listed in Table 5 for reference. Table 5Suppressed EUV CAR resist model parameters for use in rigorous modeling. These parameters were used in Sentaurus Lithography to achieve useful defectivity levels for study. The reference EUV CAR resist parameters are from a calibrated production P28 capable positive tone develop resist model and are provided to aid the understanding of modifications made to the suppressed EUV CAR model. Details of the model were published in previous work.4
NOTE: The reference EUV CAR data comes from sensitive proprietary data. Therefore, the parameters are rounded to the first significant digit. The parameters produce modeling results that are recognizable as a positive tone photo resist on a wafer. The suppressed CAR model uses parameter values necessary to image features on a simulated 0.55-NA system. The initial model was a 0.33-NA high defectivity model with physical parameters of the calibrated reference EUV model but with longer diffusion lengths, the thermalization range, and increased developer selectivity (elevated EUV CAR model4). The suppressed CAR model diffusion lengths were decreased and PAG density was increased to form images with the simulated high NA system. The elevated EUV CAR model used to develop the suppressed EUV CAR model is a contrived model based on knowledge of photoresist modeling and is intended for use in public disclosures situations in which defects are needed for study. The stochastic suppressed CAR model has a depth of focus of for line space patterns and to 40 nm for 2D patterns on a 0.55-NA scanner. A 1-mJ simulated exposure change produced a 0.1-nm space changed in a 1D structure around the 11-nm target around an 88-mJ simulated dose. 7.Appendix 2: Optical Constants Used for SimulationThe optical properties for materials used in simulation are presented in Table 6. They are taken from Sentaurus Lithography.14 Table 6Optical properties at a 13.5-nm wavelength used in this study.14
AcknowledgmentsThe following people, in no specific order, helped the authors during various phases of this study. Without their insightful and generous help, this study would not have been possible. Andreas Erdmann of Fraunhofer IISB; Peter De Bisschop, Joern-Holger Franke, Andreas Frommhold, Vicky Philipsen, Eric Hendrickx, and Kurt Ronse of IMEC; and Yudhi Kandel, Uli Klostermann, Zac Levinson, Thomas Mülders, and Ulrich Welling of Synopsys. ReferencesR. Jonckheere, III L. S. Melvin and R. Capelli,
“Stochastic printing behavior of ML-defects on EUV mask,”
Proc. SPIE, 11147 111470P https://doi.org/10.1117/12.2538153 PSISDG 0277-786X
(2019).
Google Scholar
R. Jonckheere and III L. S. Melvin,
“Stochastic printing behavior of non-local mask deficiencies in EUV lithography,”
Proc. SPIE, 11517 1151710 https://doi.org/10.1117/12.2572998 PSISDG 0277-786X
(2020).
Google Scholar
III L. S. Melvin, R. Jonckheere and E. Hendrickx,
“Two-dimensional feature stochastic printing with mask deficiencies in high-NA EUV,”
Proc. SPIE, 11609 116091S https://doi.org/10.1117/12.2584775 PSISDG 0277-786X
(2021).
Google Scholar
L. S. Melvin and R. Jonckheere,
“Contribution of mask defectivity in stochastics of EUVL-based wafer printing,”
J. Micro/Nanopattern. Mater. Metrol., 20
(2), 021003 https://doi.org/10.1117/1.JMM.20.2.021003
(2021).
Google Scholar
R. Jonckheere and L. S. Melvin,
“Contribution of mask roughness in stochasticity of high-NA EUV imaging,”
Proc. SPIE, 11854 118540I https://doi.org/10.1117/12.2601897 PSISDG 0277-786X
(2021).
Google Scholar
P. Evanschitzky and A. Erdmann,
“Advanced EUV mask and imaging modeling,”
J. Micro/Nanolithography MEMS MOEMS, 16
(4), 041005 https://doi.org/10.1117/1.JMM.16.4.041005
(2017).
Google Scholar
T. Mülders et al.,
“New stochastic post-exposure bake simulation method,”
J. Micro/Nanolithography MEMS MOEMS, 4
(4), 043010 https://doi.org/10.1117/1.2136867
(2005).
Google Scholar
J. Van Schoot et al.,
“High-NA EUVL exposure tool: key advantages and program status,”
Proc. SPIE, 11854 1185403 https://doi.org/10.1117/12.2600951 PSISDG 0277-786X
(2021).
Google Scholar
L. de Winter et al.,
“High NA EUV scanner: obscuration and wavefront description,”
Proc. SPIE, 11517 1151715 https://doi.org/10.1117/12.2572878 PSISDG 0277-786X
(2020).
Google Scholar
V. Philipsen et al.,
“Actinic characterization and modeling of the EUV mask stack,”
Proc. SPIE, 8886 88860B https://doi.org/10.1117/12.2030663 PSISDG 0277-786X
(2013).
Google Scholar
L. S. Melvin et al.,
“Applying stochastic simulation to study defect formation in EUV photoresists,”
Jpn. J. Appl. Phys., 61 SD1030 https://doi.org/10.35848/1347-4065/ac5b22
(2022).
Google Scholar
M. Matsumoto and T. Nishimura,
“Mersenne twister: a 623-dimensionally equidistributed uniform pseudo-random number generator,”
ACM Trans. Model. Comput. Simul., 8 3
–30 https://doi.org/10.1145/272991.272995 ATMCEZ 1049-3301
(1998).
Google Scholar
J.-H. Franke et al.,
“Elucidating the role of imaging metrics for variability and after etch defectivity,”
J. Micro/Nanopattern. Mater. Metrol., 21
(2), 023201 https://doi.org/10.1117/1.JMM.21.2.023201
(2022).
Google Scholar
“X-Ray interactions with matter,”
https://henke.lbl.gov/optical_constants/
(2022).
Google Scholar
BiographyLawrence S. Melvin III is a technical program manager and principal engineer at Synopsys in the Custom Design and Manufacturing Group. He has focused in areas of photolithography modeling, EUV, stochastics, application development, and resolution enhancement technologies. His current focus includes modeling stochastics in EUV systems and investigating applications to mitigate stochastic defectivity. He has collaborated to investigate the impact of EUV mask defectivity on wafer pattern stochastics since 2019. He is a senior member of SPIE. Rik Jonckheere is senior researcher of mask technology in the Advanced Patterning Process and Materials Department at IMEC. Since 1985, when he joined IMEC, he has been mainly active in the fields of e-beam lithography and mask making. He has been working on EUV reticle projects in IMECs Advanced Lithography Program since 2005. His focus is on EUV mask defectivity, its printability, and its mitigation. Since 2019, his study includes the mask contribution to stochastics of EUV-based imaging on wafers. |
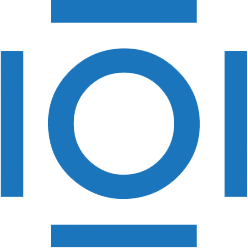
CITATIONS
Cited by 3 scholarly publications.
Photomasks
Semiconducting wafers
Stochastic processes
Critical dimension metrology
Extreme ultraviolet
Computer simulations
Optical proximity correction